Check out how to repeat a TinyML experiment on building a weather station from the new “TinyML Cookbook” and make the model smaller
Things used in this project:
Hardware components
Raspberry Pi Pico×1
Arduino Nano×1
Software apps and online services
Story
Lately, I’ve been really passionate about the field of TinyML, actively researching how to enable ML-driven solutions on low-powered devices, and I came across a newly released book, “TinyML Cookbook”, written by Gian Marco Iodice, a team and tech lead in the Machine Learning Group at Arm.
To my mind, this book is the most self-explanatory guide on TinyML existing today as the author gives a comprehensive overview of the concept in general and illustrates it with a lot of cool practical cases, so-called “recipes”. I took one of such recipes and decided to repeat the experiment of building an 8-bit model for predicting the probability of snow.
For more interest, I decided to build my model on another platform instead of the one suggested by the author (he implemented the case using TensorFlow Lite). I opted for a free-to-use platform, Neuton TinyML, in order to compare metrics of the resultant models which had to be deployable on memory-constrained MCUs (the author conducted the experiment on an Arduino Nano and a Raspberry Pi Pico).
Frankly speaking, I got quite exciting outcomes. Keep reading to learn the details :)
Original Process
The original process is described in Chapter 3 of the “TinyML Cookbook” where the author gives a detailed explanation of all the development stages of a TF-based application for an MCU, including:
- Import of weather data from WorldWeatherOnline
- Dataset preparation
- Model training with TF
- Model quantization with a TFLite converter
- Using the built-in temperature and humidity sensor on an Arduino Nano
- Using the DHT22 sensor with a Raspberry Pi Pico
- Preparing the input features for the model inference
- On-device inference with TFLu
For clarity, the process can be represented as such a scheme:
The author also provided a useful link so that everyone can easily get the source code:
Alternative Way
The example that the author gives in his book is indeed a good working case, especially since everything is described in the book to the smallest detail, and links to all the necessary resources are given.
However, I thought about how to improve the proposed workflow and reduce the number of steps by eliminating the need for quantization. Here’s the workflow that I created with Neuton TinyML:
Step-by-step Workflow
The main aim of my experiment was precisely the creation of a model to check the metrics. My workflow on the Neuton TinyML platform can be described in the following steps:
- Upload a new solution and uploaded a training dataset.
- Upload a validation dataset.
- Select a target variable “Target”.
- Choose the target metric “Accuracy”, enable the TinyML mode, and select 8-bit depth.
- Start model training.
Here are the metrics of the resultant model:
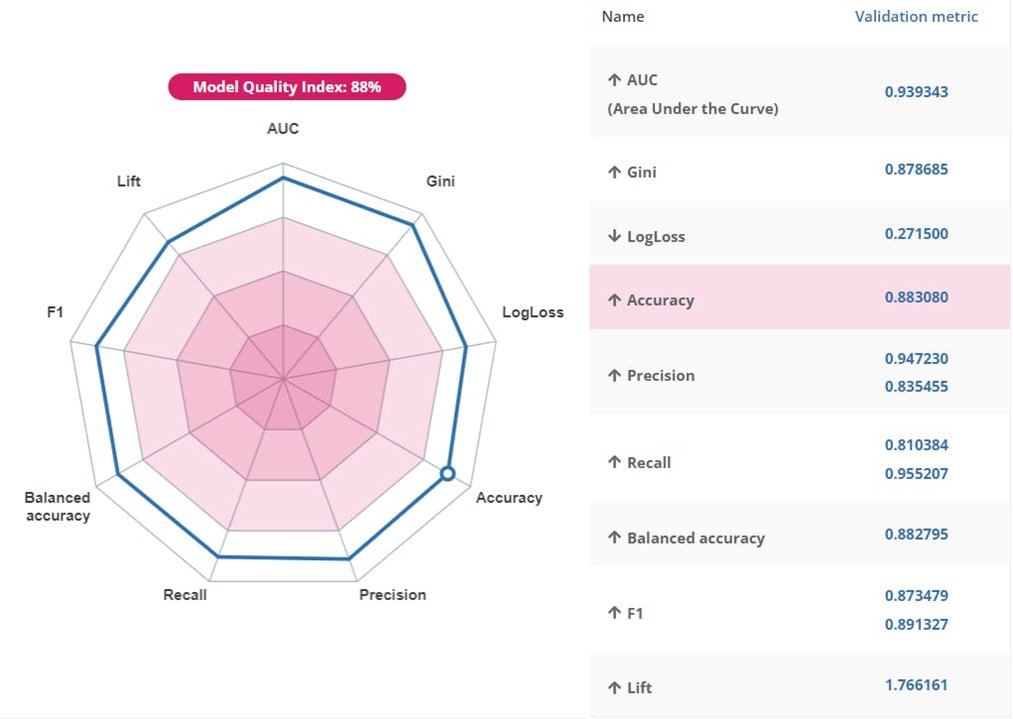
To my mind, the most noteworthy outcome that I got is that the model turned out to be super compact (0.4 Kb) without any additional compression or quantization, and the small size didn’t affect the accuracy at all (which is 88%).
If we compare the models created using the approach of the author of the book and my approach, we get the following results:
Thus, I can conclude that when using the Neuton TinyML platform, I was able to speed up the process of creating a model, as well as get better results in terms of size without compromising accuracy.
In fact, the book, “TinyML Cookbook” also describes other interesting cases, such as voice recognition, gesture-based interface building, and indoor scene classification, among others. Thank you, Gian Marco Iodice, for writing a cool book that inspires people to conduct experiments in the TinyML field.